Designing Societally Beneficial Reinforcement Learning Systems
Choices, risks, and reward reporting. Recommendations for how to integrate RL systems with society.
NOTE: An expanded version of this post can be found on the Berkeley Artificial Intelligence Research (BAIR) Blog.
I’m delighted to share a long-term project with you all charting the future where the public has a better understanding of what makes reinforcement learning (RL) both powerful and risky. This project with the Center for Long Term Cybersecurity (CLTC) and Graduates for Engaged and Extended Scholarship in Engineering (GEESE) is my long-term blogging projects turning professional. Here, I share the summary of our paper and where different parties should look.
Choices, Risks, and Reward Reports: Charting Public Policy for Reinforcement Learning Systems can be downloaded here, shared on twitter here, or a press release here.
This paper encompasses three and a half major parts.
First: a summary of what makes RL different from other types of learning (e.g. supervised and unsupervised learning), along with fundamental types of feedback it contains — control, behavioral, and exogenous.
Second: a summary of the distinct risks in this formulation, being Scoping the Horizon, Defining Rewards, Pruning Information, and Training Multiple Agents.
Third: a forward looking analysis of specific governance mechanisms and legal points of entry for RL. This is highlighted by our recommendation of documenting reward reports for any real world system.
Third and a half: an Appendix discussing cutting edge technical questions in RL research and how different guiding principles of them will define the future of data-driven feedback systems.
This paper centers around the types of feedback central to the RL framework and a specific set of risks RL design manifests. Here I detail them to give a primer for further reading.
Types of feedback:
Control Feedback: the classic notion of feedback from linear systems where the action taken depends on the current measurements of the system.
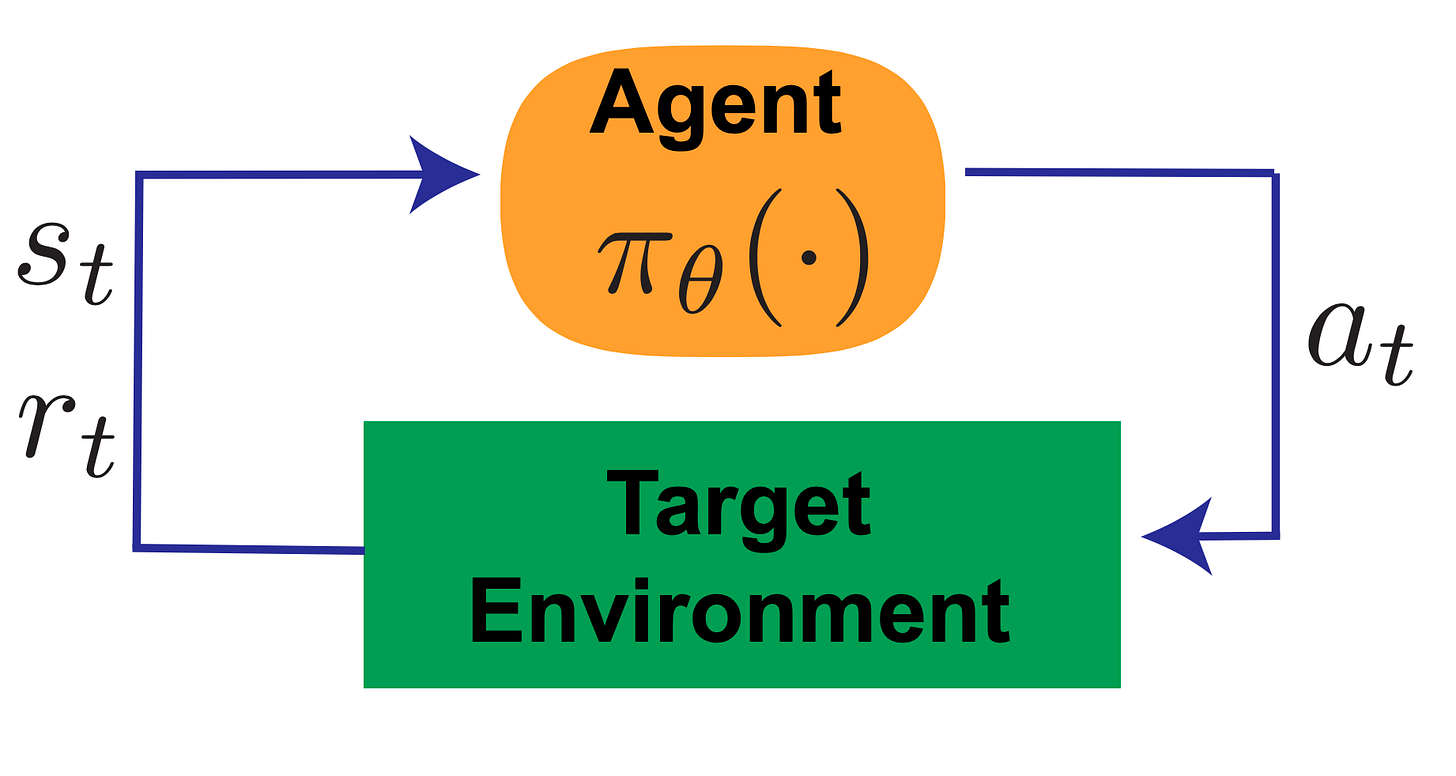
Behavioral Feedback: the often-defining feature of RL, trial and error learning and how that evolves over time.
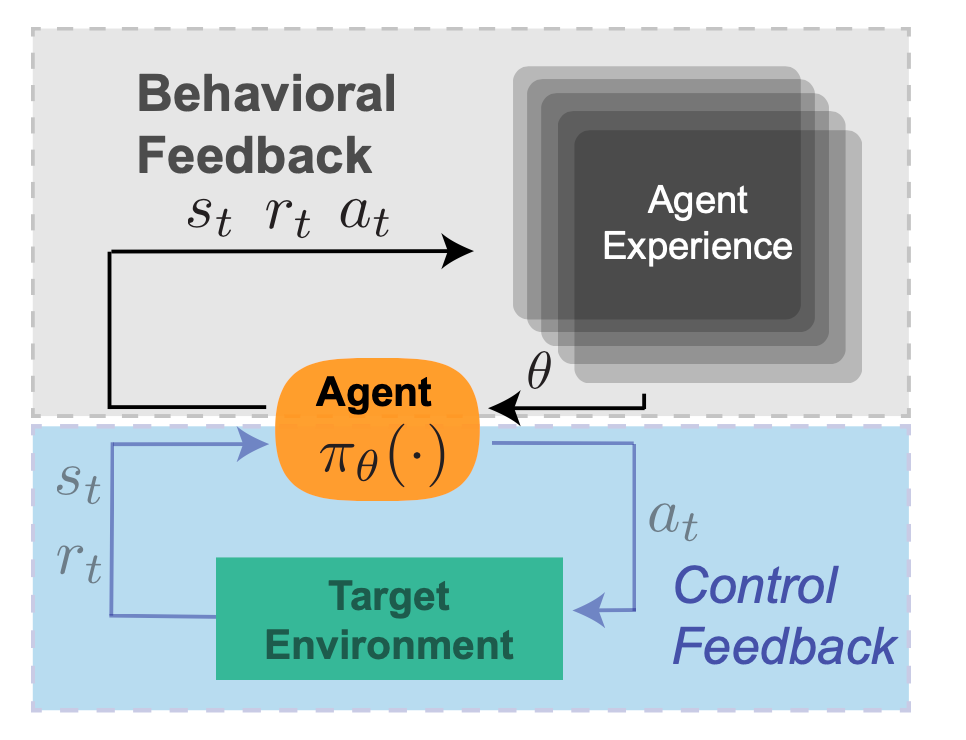
Exogenous Feedback: the future purview of RL designers — how a optimized environment impacts systems outside of the predetermined domain.
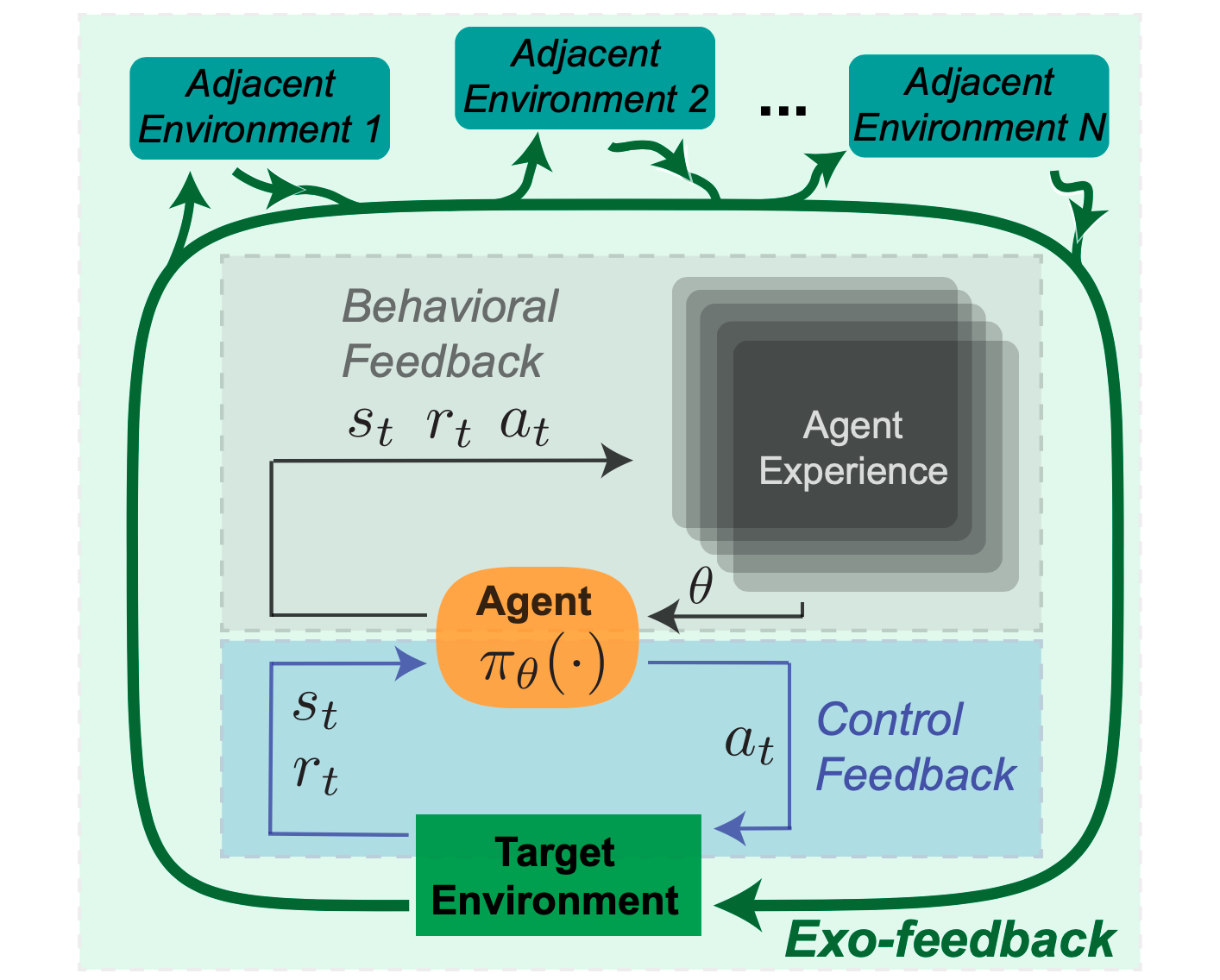
Types of risk:
Scoping the Horizon: determining the timescale of an agents goals has an incredible impact on behavior. In research this is often discussed in the realm of sparse rewards, but in the real world agents can externalize costs depending on the defined horizon.
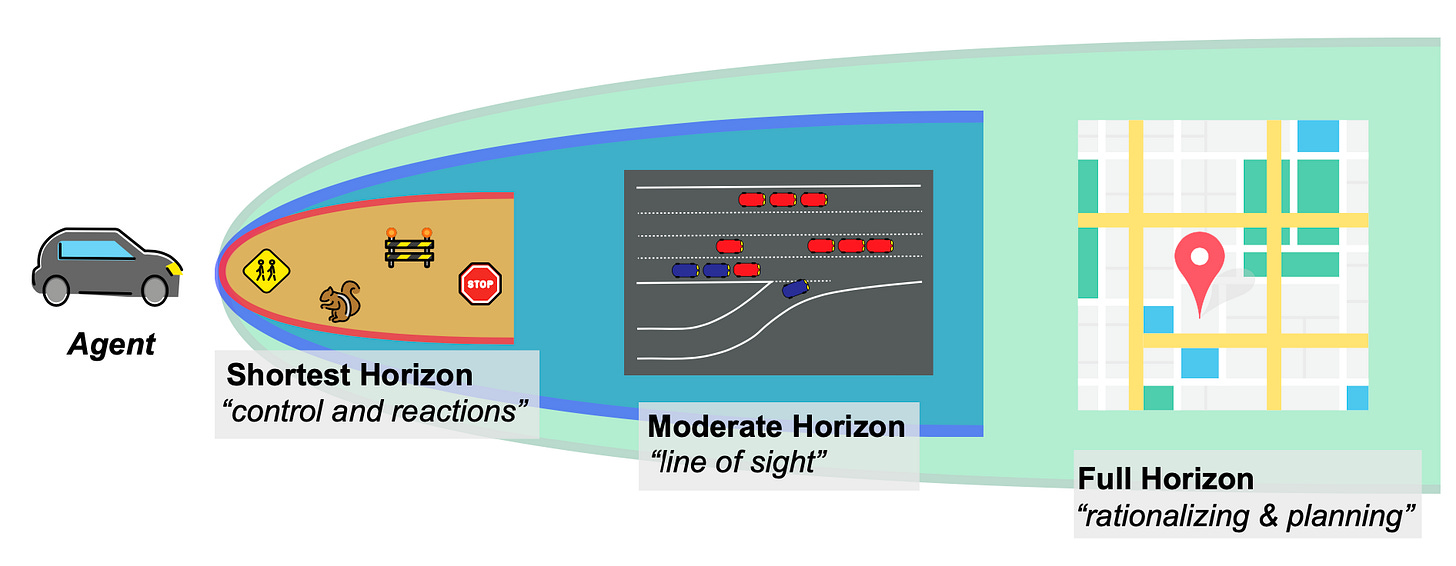
Defining Rewards: the classic risk of RL systems, reward hacking, where the designer and agent negotiate behaviors based on a specific function. In the real world, this can often result in unexpected and exploitative behavior.
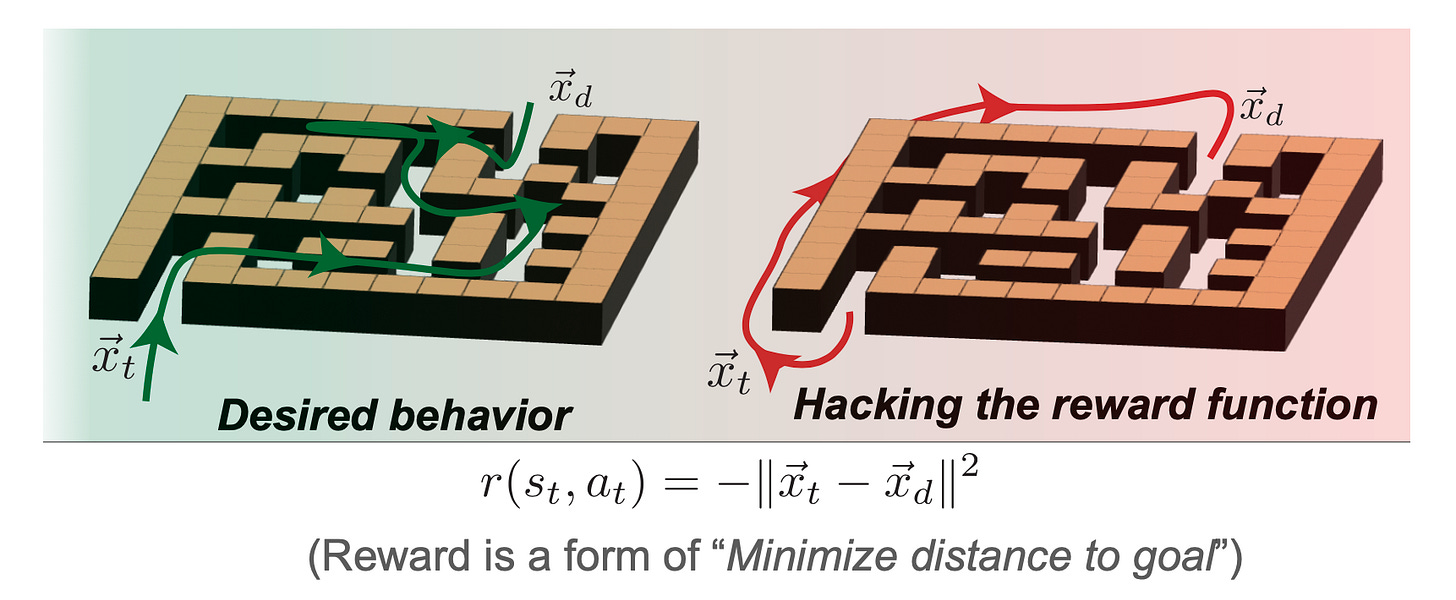
Pruning Information: a common practice in RL research is to change the environment to fit your needs. In the real world, modifying the environment is changing the information flow from the environment to your agent. Doing so can dramatically change what the reward function means for your agent and offload risk to external systems.
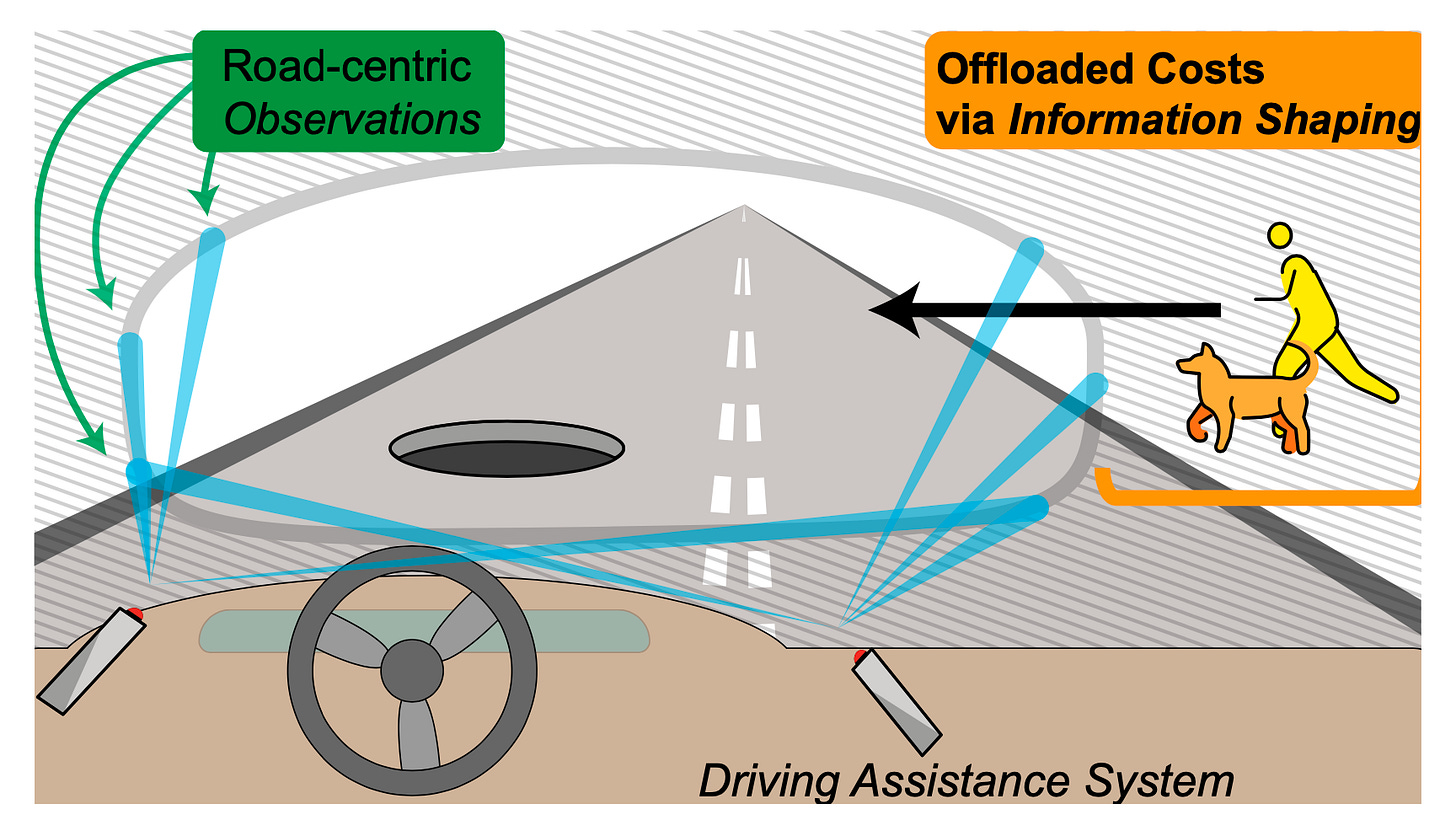
Training Multiple Agents: little is known how learning systems will interact. When their relative concentration increases, the terms defined in their optimization can re-wire norms and values encoded in the application domains.
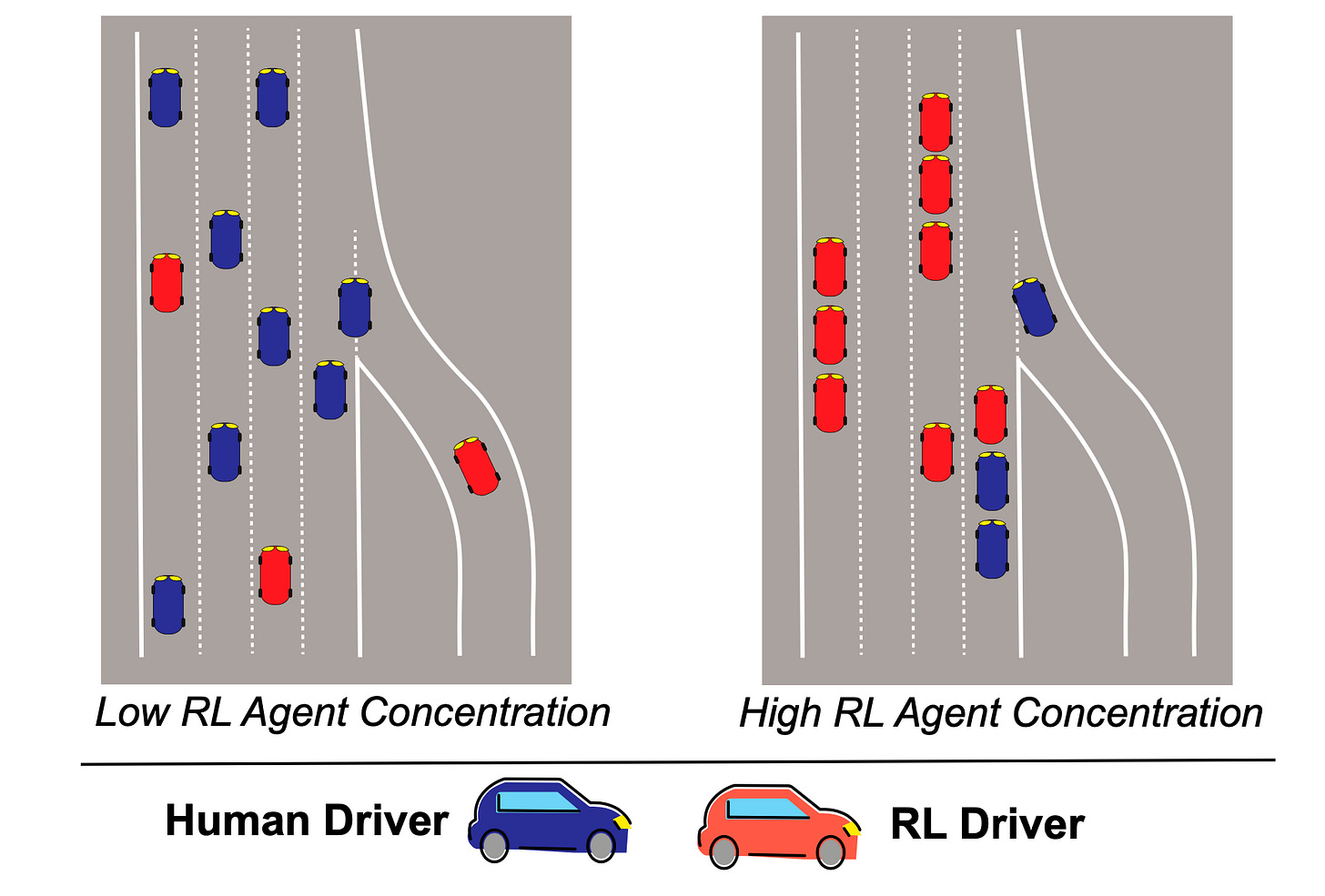
The TL;DR - Reward Reporting
We propose Reward Reports, a new form of documentation that foregrounds the societal risks posed by automated decision-making systems (ADS), whether explicitly or implicitly construed as RL. Building on proposals to document datasets and models, we focus on reward functions: the objective that guides optimization decisions in feedback- laden systems. Reward Reports comprise questions that highlight the promises and risks entailed in defining what is being optimized in an AI system, and are intended as living documents that dissolve the distinction between ex- ante specification and ex-post harm. As a result, Reward Reports provide a framework for ongoing deliberation and accountability after a system is deployed.
(more forthcoming on this soon)
Where do I start?
Here I provide some guidance on where you should start if you have different backgrounds and goals around RL systems:
I am a technical expert looking to understand the risks...
The most substantial section of our paper, “A Topology of Choices and Risks in RL Design” will translate common practice of RL engineers and researchers into clear mechanisms for action that impact the domain of interest and users.
I am interested in learning what RL really encompasses...
Start from the beginning! The “Introduction” gives an excellent overview on what makes RL click. This was one of the most enjoyable sections to create and would be very useful material for any introductory AI course.
I have a deep understanding of RL but have not thought about applying it to critical domains...
We have a specific section dedicated to walking through how risks emerge in three application domains: social media recommendations, vehicle transportation, and energy infrastructure.
I am a policymaker looking for your recommendation...
Honestly, we hope you read the whole thing, but the governance mechanisms can give you a TL;DR on the necessary actions.
I am curious on your thoughts of the future of RL research...
The appendix it is. Explore ideas such as how offline RL breaks feedback loops and where the model-based vs. model-free debate will engage with society.