What is ML for Climate Change?
A new “subfield” founded in 2019 is making waves, and is more accessible than I first thought.
In this issue, with the devolving world order, seeking positive, engaging work, I wanted to learn a) what actually is machine learning for climate change and b) are there reasonable paths for us to dive in and contribute? To quote the call for action in a paper I cite heavily later:
Groundbreaking technologies have an impact, but so do well-constructed solutions to mundane problems.
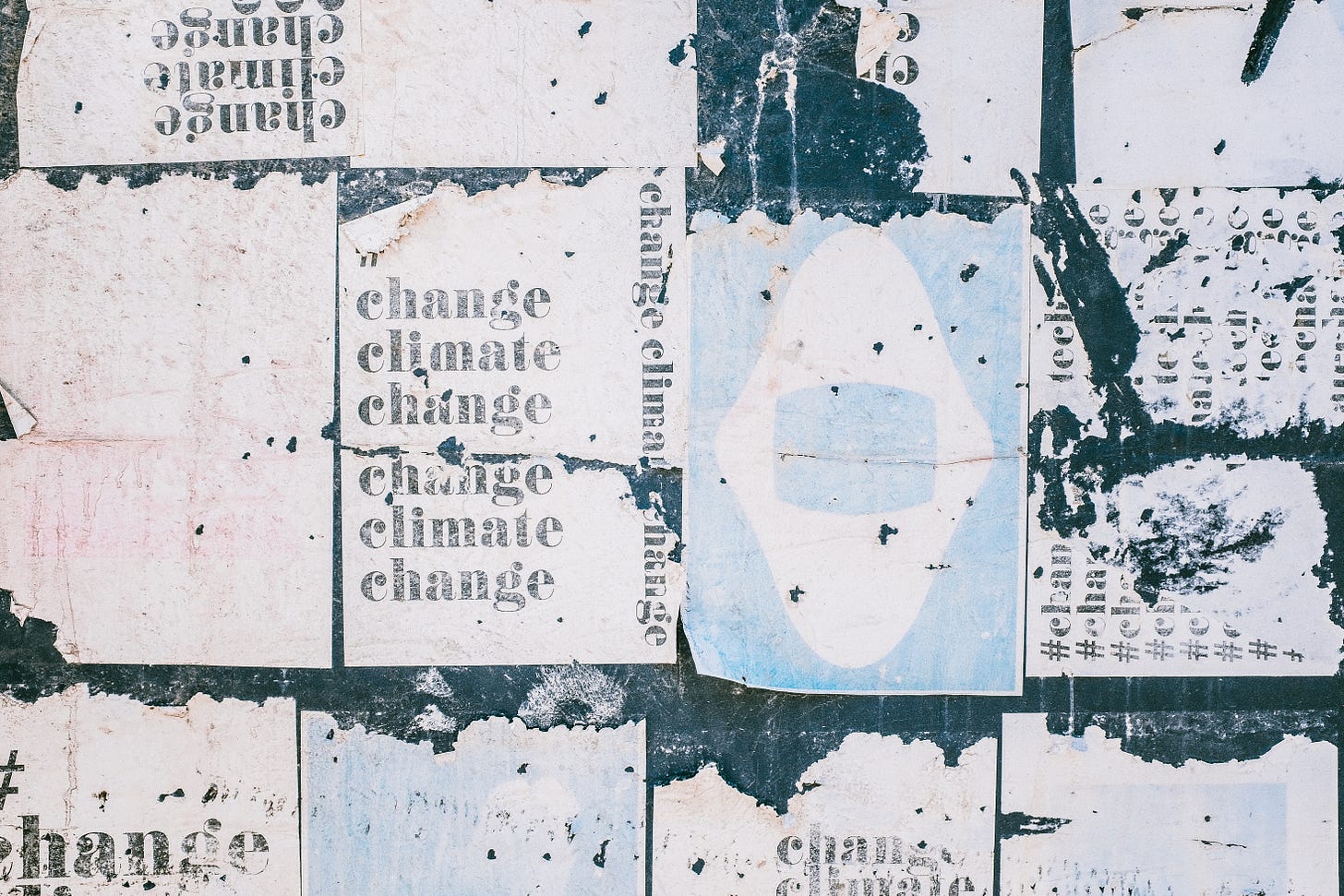
Recent work
To start with, I knew there were a bunch of recent workshops on climate change and machine learning (such as ICLR 2020, ICML 2019, NeurIPs 2019 editions). When looking here, it turns out it is a centralized group of climatechange.ai. This seems good to me, but I was hoping to learn what people are actually working on.
I wrote a little script to scrape the titles and authors of the workshop proceedings and came up with this list of keywords (learned about NLP and stop words along the way).
LEARNING, USING, CLIMATE, DEEP, MACHINE, DATA, NETWORKS, CHANGE, SATELLITE, PREDICTION, IMAGERY, NEURAL, WEATHER, POWER, FORECASTING, ENERGY, TOWARDS, MODELS, CARBON, REINFORCEMENT, BASED, DETECTION, ENVIRONMENTAL, MONITORING, FLOW, VIA, DYNAMICS, FRAMEWORK, SOLAR, RISK, CLOUD, GRID, LEARNING-BASED, FOREST, CONSERVATION, SMART, ANALYSIS, OPTIMAL, MAPPING, URBAN, INTELLIGENCE, RENEWABLE
I expected some buzzword-ness, but this was pretty much a non-entity in terms of teaching me what people are working on. Some keywords that provide insight may be this subset (remove data and learning description words):
NETWORKS, SATELLITE, WEATHER, POWER, ENERGY, FLOW, SOLAR, DYNAMICS, GRID, FOREST, CONSERVATION, MAPPING, URBAN
It reads as a list of applications in the space of urban development, power systems, energy grids, conservation, and dynamic systems. After this cursory analysis, I realized I actually needed to read the 100page white paper initializing the field.
I am putting the data for paper information here. You can register for the 2020 Virtual NeurIPs conference for $100 ($25 student) and attend the next iteration on the workshop for Tackling Climate Change with Machine Learning.
Tackling Climate Change with Machine Learning — Seminal Paper
This paper from 22 authors at 17 institutions preludes the workshops I mentioned and provides detailed explanations on where people can start. If you get nothing else out of this blogo-post, get this: machine learning for climate change is a movement encouraging application-based machine learning research that’ll benefit the planet’s long-term ecosystem (not to say all the changes will take a long time).
I’ll leave the paper to summarize the potential application areas, and the reader to open to document if they are interested in learning more:
ML can enable automatic monitoring through remote sensing (e.g. by pinpointing deforestation, gathering data on buildings, and assessing damage after disasters). It can accelerate the process of scientific discovery (e.g. by suggesting new materials for batteries, construction, and carbon capture). ML can optimize systems to improve efficiency (e.g. by consolidating freight, designing carbon markets, and reducing food waste). And it can accelerate computationally expensive physical simulations through hybrid modeling (e.g. climate models and energy scheduling models).
Essentially, all the application spaces of remote sensing, scientific discovery, energy-intensive systems, and more have many data that is not being touched, and machine learning has proven extremely useful at finding patterns in any big dataset. I separate this point from the list to make it clear — computer scientists alone cannot solve these problems:
meaningful action on climate problems requires dialogue with fields within and outside computer science and can lead to interdisciplinary methodological innovations, such as improved physics-constrained ML techniques.
The machine learning tools developed in the last decade have had impressive results in distilling patterns from data in so many fields. While there are still many reasons to be skeptical of techniques, there are good reasons that some new tools will work in some of these areas. For example, reinforcement learning, which is generally accepted to be unstable, hard to transfer to the real world, and full of un-modeled behavior is simultaneously very useful in power grid optimization (example 1, example 2). Why does reinforcement learning work here: the system is grounded in very well-known physics and constrained variables — so when the RL agent does something weird, it can easily be flagged as not physically possible, and so on (I am starting a reading group with some graduate students going into the differences that make safe or unsafe real world RL applications, so if you are an expert and want to talk, or are interested, let me know).
There is a caveat though, which is crucial considering how to deploy this technology: many countries don’t have the data infrastructure that underpins so many industries in the U.S. The paper gives the example of different data-logging at energy plants from the U.S. to India — this turns into a second machine learning problem, namely transfer learning after figuring out how to optimize the American grid.
They provide a call to action, which is a good summary of how I now feel about it.
Learn. Identify how your skills may be useful – we hope this paper is a starting point.
Collaborate. Find collaborators, who may be researchers, entrepreneurs, established companies, or policy makers. Every domain discussed here has experts who understand its opportunities and pitfalls, even if they do not necessarily understand ML.
Listen. Listen to what your collaborators and other stakeholders say is needed. Groundbreaking technologies have an impact, but so do well-constructed solutions to mundane problems.
Deploy. Ensure that your work is deployed where its impact can be realized.
If you are curious where your expertise may overlap, look at this grid. I think we have an obligation to solve the mundane problems, because they tend to be low hanging fruit. Machine learning practitioners can look at the top and see where their skills are useful, then reference the original paper. Everyone else can look at the left, and then it will pinpoint what ML tools are most useful to learn and leverage in your field.
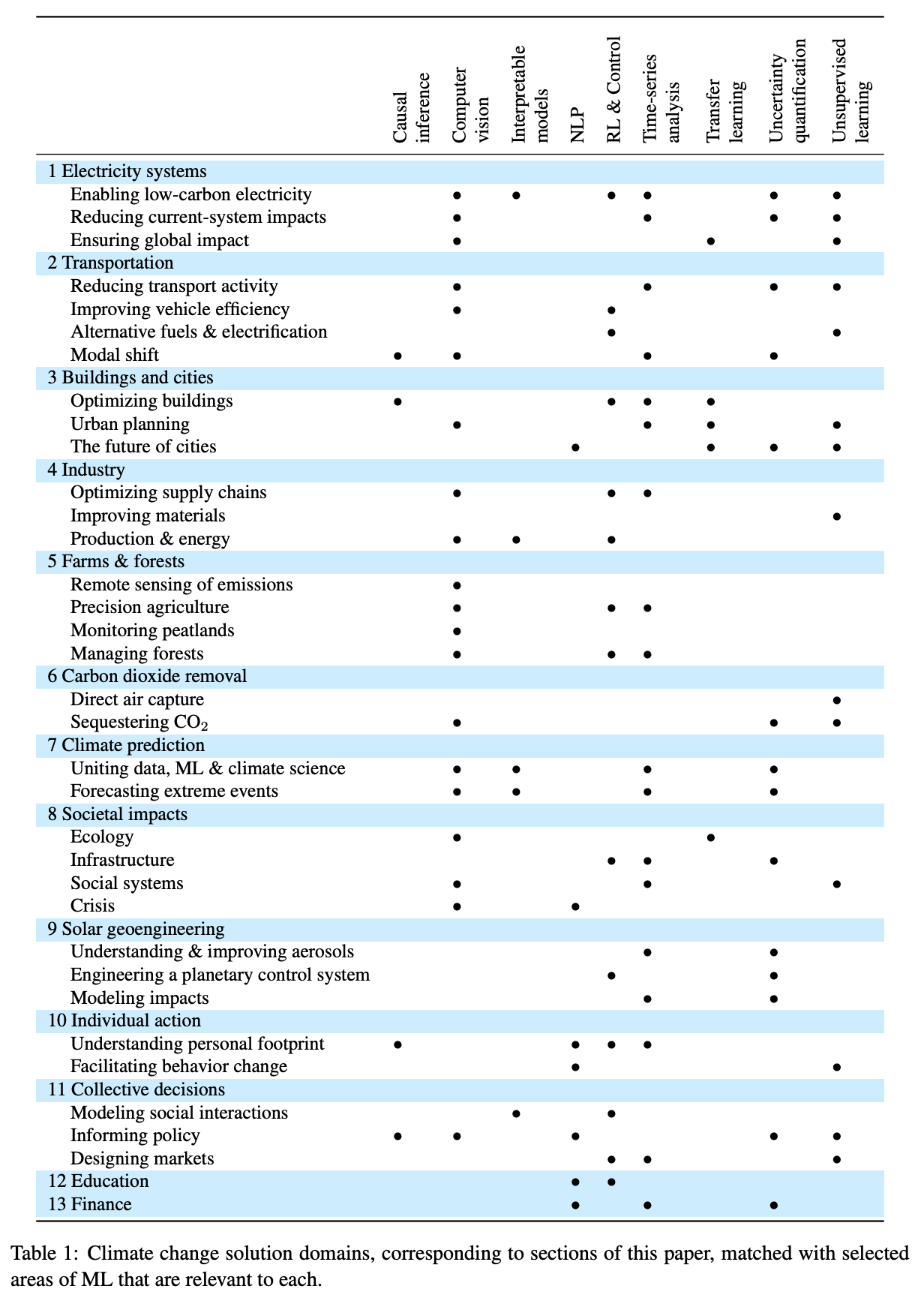
Systematic Changes to Encourage Participation
How can we encourage researchers to team up with practitioners to solve the mundane problems mentioned in the paper. I know my advisor would be totally onboard for me doing this, but the coverage of advisors and managers is not uniform. I have talked about the academic system being a tad broken in terms of incentives, but I want to add another variable to the equation: how to encourage societally beneficial work that is pretty much unnoticed by many academics (fewer citations, bleh).
Are people at Google Brain and Facebook AI incentivized to work on this? Should I spend 6 months working in this space as an independant researcher to stake some territory? I just want to open the discussion so people think about what actually is limiting spending time in the area.
Creating Technology as an Alternate to Studying Climate Change
Another thought I always throw into the discussion on climate change is what technology can we make that’ll either a) change the public perception of “green” devices or b) will benefit the environment without the consumer caring (it’s just better a la Tesla). My impression from the paper above is that the problems of mitigating harm, optimizing current infrastructure, and measuring impacts of current systems are way lower barrier to enter. I still hold out that making mindset shifting products is long term more beneficial. Here are some statistics from Tesla and their report from last year.
Where on the spectrum of out-innovate climate change versus mitigate climate change through public perception campaigns and conservation do you fall?
See you at the next iteration on the workshop for Tackling Climate Change with Machine Learning, either December 11th or 12th 2020.
Want to support this Democratizing Automation experience and more high-signal content on AI, robotics, and automation?
Click the share button and send it to one of your buds.
Tap the cute heart button at the bottom — making an account lets you comment too!
Should I start a podcast? I think it’s a matter of when, if not if.
Talk to me here (Substack), here @natolambert, or here.