It's 2024 and they just want to learn
The state of the ML communities big and small starting 2024. My general expectations for the year.
Going into 2024 feels like a breath of fresh air compared to 2023 because we know what we’re going to get in the ML world. We know we are going to get tons of breakthroughs. We know some of the advances will come from known entities and some will come from new ones. We know that we cannot predict the intricacies. We know the models work. What we don’t know is: Whose narrative will dominate the progress and momentum we’re feeling in 12 months time?
Last year this time we were starting to understand that ChatGPT was the biggest event in our field since AlexNet, last spring everyone realized that the next few years were going to be a race, and through the rest of the year everyone finished hiring their founding team and joining organizations that fit their goals.
This year we’ll almost surely get all of the following large language models (LLMs): Llama 3, Mistral-Medium, the next OpenAI model (they won’t call it GPT5 because it’ll mostly use the same architecture), Gemini Ultra, a long slew of fine-tunes, and much more. Many of these will come in the first 6 months of the year.
2024 is going to be a year of rapid progress in capabilities and robustness as the industry spends some of the time it has to show which LLM products and services will back up the vast amounts of investment that have flowed in in the last 18 months.
If you compare your recent work to your progress baseline from 12 months ago, the pace we are iterating at is easily 10 times higher for most people. Many people will feel like they’re not even comparable because they changed from exploring to building. Last January looked like planning teams, considering $100k-$10mil data contracts, figuring out the basics of LLM techniques like reinforcement learning from human feedback (RLHF) and instruction fine-tuning (IFT), axing idling teams, and much more corporate maneuvering.
Now, it’s all release schedules for projects nearing completion and planning 6 to maybe 18 months out (for the most ambitious projects).
In the last year, we’ve also seen the expectations of who is in power and who drives the narrative shift entirely. In the eras of opportunity, it is when new figures make their name and dwarf those who they previously thought were peers. It’s not too late to have that level of impact in LLMs, you’re still maybe even early.
This is the new normal for working in machine learning and language models. Jonathon Frankle mentioned on the Latent Space recap that NeurIPs was the most normal it’s seemed in years. This is because people have either accepted their lane or accepted the fight it takes to expand the scope of their worldview. No more big lab hate and no more academic copium; it’s time to cook. It’s time to prove yourself and your beliefs.
There’s a famous meme or saying that’s been going around the last few weeks again about training large models: they just want to learn. All anthropomorphization comments aside (it’s clear that models do not want anything innately right now), it’s true. It’s the best way to describe the low-level feeling on the ground in LLMs. If you go at tasks with a few levers (combinations of data and training platforms), abundant motivation, and especially scale, the signal almost always comes. The idea is that there’s something deeply aligned in how we’re training models and structuring our data, but that philosophical digression on whether scaling works is for another day.
I find it somewhat unsettling, but the simple idea of compute plus data equals intelligence can take people a long way.
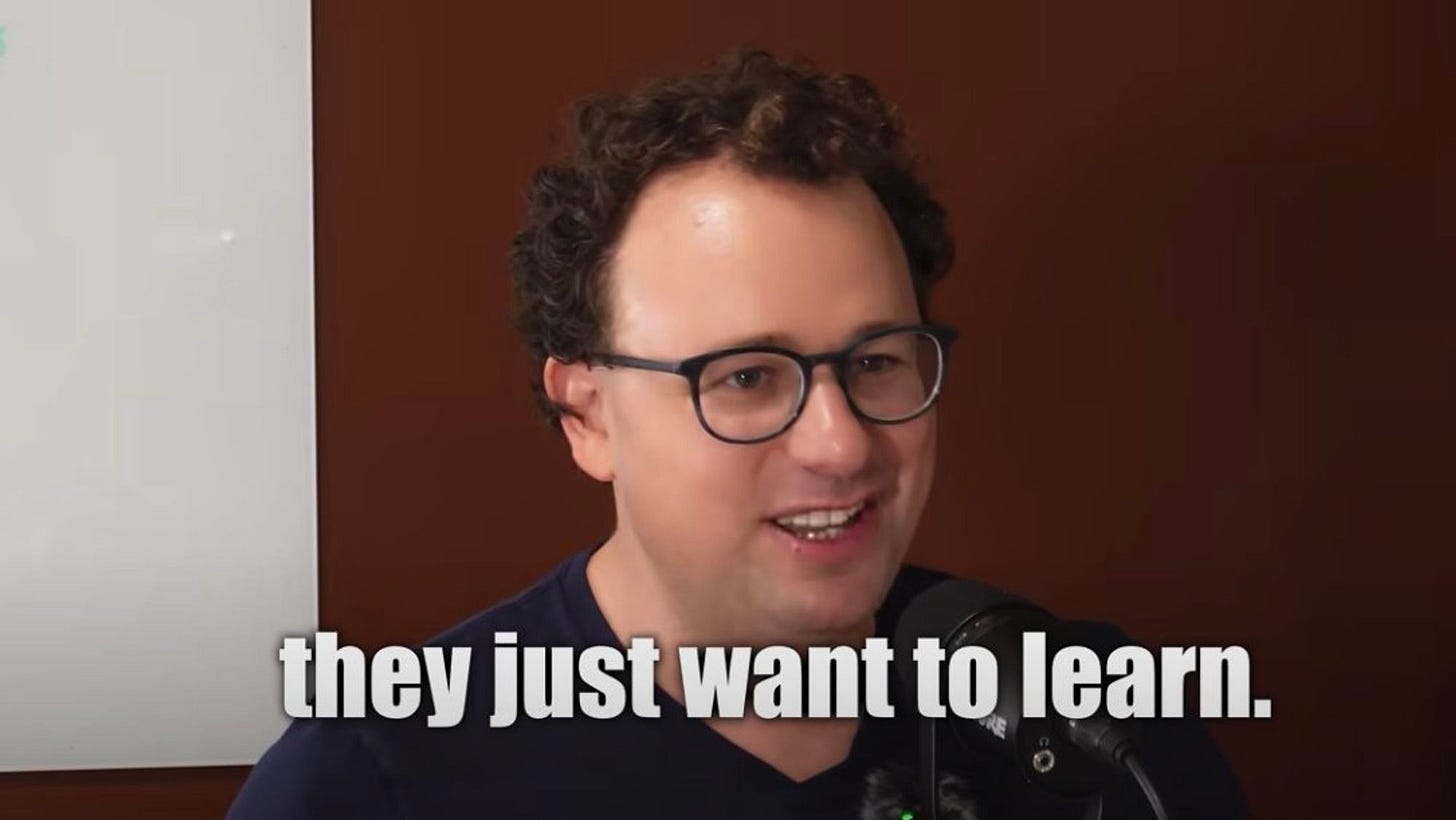
This was epitomized for me in 2023 with the fact that our Tulu 2 70B model (which was state-of-the-art in ChatBotArena for a period) was our first training run! The environment where the first training run can work favors action and consistency motivation more than anything else.
If you don’t like working like this, that’s fine, but hopefully, you can at least find it entertaining.
Tribes, E/Science, and the third rail
While everything is on track across multiple communities, that also unlocks the ability for people to tap into excitement and energy that they’ve never experienced in their career (and maybe lives). The last I felt anything remotely close to this was my four years as a lightweight rower at Cornell. I wouldn’t normally mention this, but the overlap is remarkably similar. It feels like showing up every day with all of your chips on the table. Intensity and expectations for yourself are extremely high. Once consistent milestones start flowing, they get easier and easier.
The situation where people feel like they have near unlimited energy and motivation to march towards their goals only comes from being in an environment where belief is encouraged, community is fostered, and opportunity is available. ML has all of these ingredients for believers across many different tribes and it’s enthralling to watch. I call this “tapping into the third rail” of life, and it’s easily recharged with the regular chaos and quagmire of bad takes that is Twitter. I see many people who are obviously in this state.
I don’t agree with every tribe, but I respect people who are willing to tap into the energy overload and try and make it real. It can be life-consuming, it can come with wild ups and downs, and it can lead you astray, but at the end of the day, there’s something immensely human about it. In some ways, the same goes for communities as goes for models, they want to build. The train tracks are set and they’re en route.
I encourage people to go out and follow the path they’re dreaming but encourage them to not let the closed-mindedness that often comes with it consume them. Given how fast the field is moving, it should be fairly obvious that almost everyone has huge error bars on all of their propositions. It’ll affect you in ways you don’t expect, but at the end of the day, the most passionate people in the LLM space are generally those who deeply care about how ML will interface with society. Many people describe the methods for achieving similar goals in ML in different ways. Those purely searching to make their buck don’t have the staying power to build for the 2-4 years that it’ll take for the dust to even start to settle.
A lot of people across these groups feel like everything they do or build is moving their goals and vision one step closer to reality. A core tendency and strife I see in the ML community is the fracture between builders and critics. A core value of mine is that the way to enable better systems for society is to build value-aware systems that people want to use. I find that especially the accelerationist and AI safety communities get criticisms from others that land as “your line of work is bad” rather than “this is how you improve your line of work.” 2024 is the time for builders. Builders love to listen to builders – show the tradeoff that you’re advocating for if you think a method is causing harm to a group or ideal. It’s also just more fun to build something than it is to criticize other peoples’ works, so that’s why so many critics will be shrugged off, the targets want to get back to their projects.
Going somewhere where I can tap into this energy source and progress the science of LLMs as far as we can in public is ultimately why I joined the Allen Institute for AI – there’s alignment from the top to bottom to promote the common good by building and understanding language models. There’s no venture capital or shareholder misalignment that can mitigate this from becoming a reality.
Until concrete safety risks are demonstrated, I see far more harm coming from market capture and insufficient analysis of the models. I don’t expect 2024 to be the year where we see a minor disaster that gives much more credence to the AI Safety ideals because the models are not yet deeply integrated in or as compute infrastructure. Before LLMs get to this point, we should deeply study their failure modes and tendencies to be led astray. This likely comes with developing many new safety and monitoring practices that need access to things like weight updates, activations, and robustness statistics that are not available behind lab doors. Building models is the way to do this learning and demonstrate to broader stakeholders that it is needed.
While many tribes are well known and have their own manifestos, like techno-optimism / accelerationists (E/ACC), AI Safety (doomers), AGI theocrats, and fairness & ethics (the most prominent three), I see many more splinters like the techno-pragmatists and the AI optimists.
As so much of open source’s weight has been shifting towards the E/ACC community, I’ve felt less of a home there. For me and many scientists, the goal is not velocity. The goal is understanding, building good systems, and acting with clear principles and values. I’m hoping to mobilize more participation among scientists who have similar views but haven’t quite worked up the energy to dive into the discourse. It’s time for the open science group to make their voices heard (which is part of why I’ve started doing interviews). Regardless, I have many close friends across all of the communities and will do my best to celebrate your successes as well.
Good luck with your adventures for the year!
Audio of this post will be available later today on podcast players, for when you’re on the go, and YouTube, which I think is a better experience with the normal use of figures.
Newsletter stuff
Models
Argilla, who joined the Zephyr and Tulu trend with Notus, released a DPO’ed Mixtral model.
MosaicBERT studies the best ways to serve BERT models (popular encoder-only models) in 2024. Paper here, models here.
A continued-training base model with Thai capabilities crossed my paths. Seems interesting as a short term solution to multilinguality.
Some great documents tracking LLMs from Stella Biderman of Eluether.
1. Common hyparam settings for leading LLMs.
2. A list of all notable LLMs.A paper released models for “low data alignment,” which seems like the DPO paper a la LIMA. https://github.com/hkust-nlp/deita
Links
A good piece of commentary on the NYT v. OpenAI thingy.
Another Sasha Rush talk, this time on moving away from Attention for diffusion models too! Normal diffusion models downsample to attention via UNets or patches (as dense networks are important for the image models)
An interesting intuition of deep learning from John Schulman.
A thread on Twitter got big on the topic of “DPO mastery."
A high effort robot + LLMs summary for 2023 from
(friend of the pod):
Housekeeping
New paid feature, Discord: an invite to the subscriber-only Discord server is in email footers.
Interconnects referrals: You’ll accumulate a free paid sub if you use a referral link from the Interconnects Leaderboard.
Student discounts: Want a large paid student discount, go to the About page.